Theme lead
Publications by theme lead
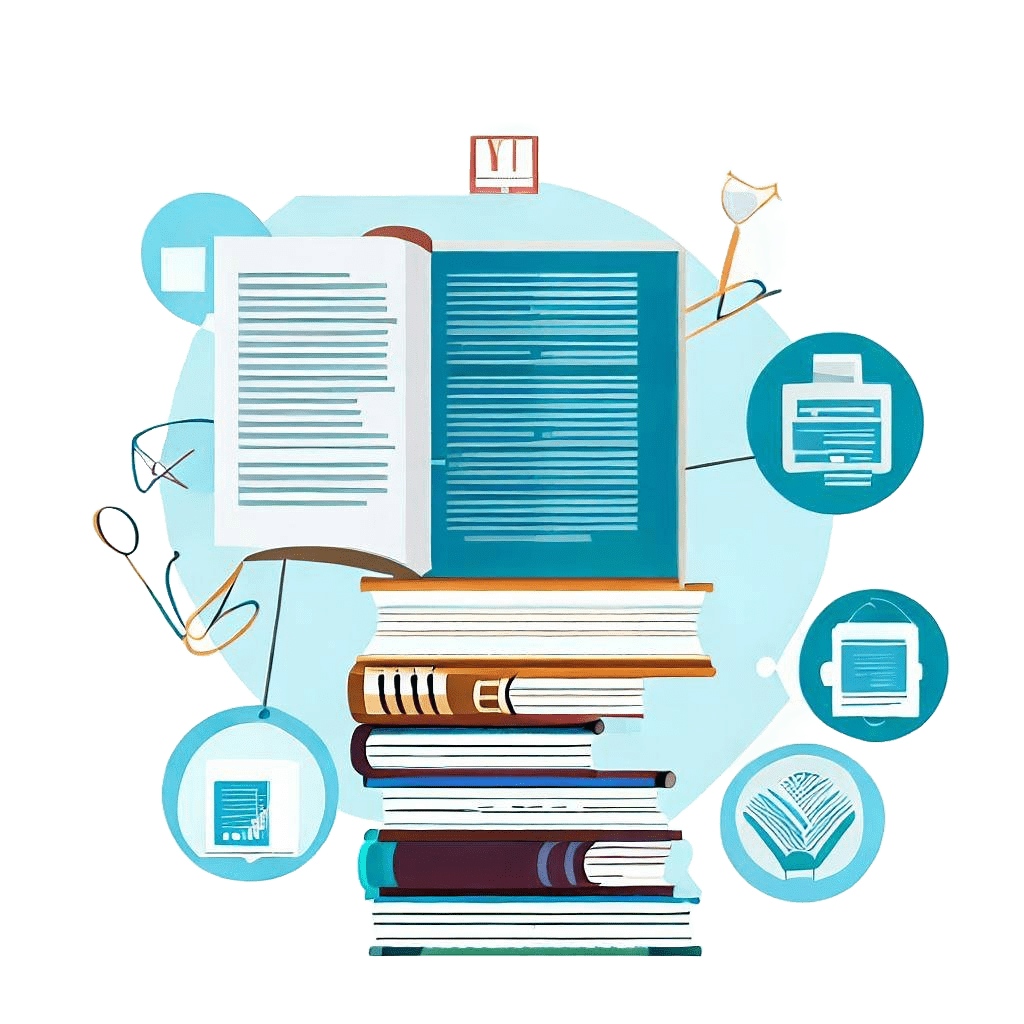
Recent publications
A novel peptide-based tau aggregation inhibitor as a potential therapeutic for Alzheimer's disease and other tauopathies
Aggidis A, Devitt G, Zhang Y, Chatterjee S, Townsend D, Fullwood NJ, Ortega ER, Tarutani A, Hasegawa M, Cooper A, Williamson P, Mendoza-Oliva A, Diamond MI, Mudher A and Allsop D
A novel peptide-based tau aggregation inhibitor as a potential therapeutic for Alzheimer's disease and other tauopathies
Aggidis A, Devitt G, Zhang Y, Chatterjee S, Townsend D, Fullwood NJ, Ortega ER, Tarutani A, Hasegawa M, Cooper A, Williamson P, Mendoza-Oliva A, Diamond MI, Mudher A and Allsop D
As aggregation underpins Tau toxicity, aggregation inhibitor peptides may have disease-modifying potential. They are therefore currently being designed and target either the VQIVYK aggregation-promoting hotspot found in all Tau isoforms or the VQIINK aggregation-promoting hotspot found in 4R isoforms. However, for any Tau aggregation inhibitor to potentially be clinically relevant for other tauopathies, it should target both hotspots to suppress aggregation of Tau isoforms, be stable, cross the blood-brain barrier, and rescue aggregation-dependent Tau phenotypes in vivo.
Pathways to Alzheimer's Disease: The Intersecting Roles of Clusterin and Apolipoprotein E in Amyloid-β Regulation and Neuronal Health
Laslo A, Laslo L, Arbănași EM, Ujlaki-Nagi AA, Chinezu L, Ivănescu AD, Arbănași EM, Cărare RO, Cordoș BA, Popa IA and Brînzaniuc K
Pathways to Alzheimer's Disease: The Intersecting Roles of Clusterin and Apolipoprotein E in Amyloid-β Regulation and Neuronal Health
Laslo A, Laslo L, Arbănași EM, Ujlaki-Nagi AA, Chinezu L, Ivănescu AD, Arbănași EM, Cărare RO, Cordoș BA, Popa IA and Brînzaniuc K
One of the hallmarks of Alzheimer's disease (AD) is the deposition of amyloid-β (Aβ) within the extracellular spaces of the brain as plaques and along the blood vessels in the brain, a condition also known as cerebral amyloid angiopathy (CAA). Clusterin (CLU), or apolipoprotein J (APOJ), is a multifunctional glycoprotein that has a role in many physiological and neurological conditions, including AD. The apolipoprotein E (APOE) is a significant genetic factor in AD, and while the primary physiological role of APOE in the brain and peripheral tissues is to regulate lipid transport, it also participates in various other biological processes, having three basic human forms: APOE2, APOE3, and APOE4. Notably, the APOE4 allele substantially increases the risk of developing late-onset AD. The main purpose of this review is to examine the roles of CLU and APOE in AD pathogenesis in order to acquire a better understanding of AD pathogenesis from which to develop targeted therapeutic approaches.
Parasagittal dural volume correlates with cerebrospinal fluid volume and developmental delay in children with autism spectrum disorder
Agarwal N, Frigerio G, Rizzato G, Ciceri T, Mani E, Lanteri F, Molteni M, Carare RO, Losa L and Peruzzo D
Parasagittal dural volume correlates with cerebrospinal fluid volume and developmental delay in children with autism spectrum disorder
Agarwal N, Frigerio G, Rizzato G, Ciceri T, Mani E, Lanteri F, Molteni M, Carare RO, Losa L and Peruzzo D
The parasagittal dura, a tissue that lines the walls of the superior sagittal sinus, acts as an active site for immune-surveillance, promotes the reabsorption of cerebrospinal fluid, and facilitates the removal of metabolic waste products from the brain. Cerebrospinal fluid is important for the distribution of growth factors that signal immature neurons to proliferate and migrate. Autism spectrum disorder is characterized by altered cerebrospinal fluid dynamics.
Defining an Ageing-Related Pathology, Disease or Syndrome: International Consensus Statement
Short E, Adcock IM, Al-Sarireh B, Ager A, Ajjan R, Akbar N, Akeroyd MA, Alsaleh G, Al-Sharbatee G, Alavian K, Amoaku W, Andersen J, Antoniades C, Arends MJ, Astley S, Atan D, Attanoos R, Attems J, Bain S, Balaskas K, Balmus G, Bance M, Barber TM, Bardhan A, Barker K, Barnes P, Basatemur G, Bateman A, Bauer ME, Bellamy C, van Beek E, Bellantuono I, Benbow E, Bhandari S, Bhatnagar R, Bloom P, Bowdish D, Bowerman M, Burke M, Carare R, Carrington EV, Castillo-Quan JI, Clegg P, Cole J, Cota C, Chazot P, Chen C, Cheong Y, Christopher G, Church G, Clancy D, Cool P, Del Galdo F, Dalakoti M, Dasgupta S, Deane C, Dhasmana D, Dojcinov S, Di Prete M, Du H, Duggal NA, Ellmers T, Emanueli C, Emberton M, Erusalimsky JD, Feldmeyer L, Fleming A, Forbes K, Foster TC, Frasca D, Frayling I, Freedman D, Fülöp T, Ellison-Hughes G, Gazzard G, George C, Gil J, Glassock R, Goldin R, Green J, Guymer R, Haboubi H, Harries L, Hart S, Hartley D, Hasaballa S, Henein C, Helliwell M, Henderson E, Heer R, Holte K, Idris I, Isenburg D, Jylhävä J, Iqbal A, Jones SW, Kalaria R, Kanamarlapudi V, Kempf W, Kermack AJ, Kerns J, Koulman A, Khan AH, Kinross J, Klaucane K, Krishna Y, Gill HS, Lakatta E, Laconi E, Lazar A, Leeuwenburgh C, Leung S, Li X, van der Linde I, Lopes LV, Lorenzini A, Lotery A, Machado P, Mackie S, Madeddu P, Maier A, Mukkanna K, Manousou P, Markey O, Mauro C, McDonnell B, Medina RJ, Meran S, Metzler-Baddeley C, Meglinksi I, Milman N, Mitteldorf C, Montgomery R, Morris AC, Mühleisen B, Mukherkee A, Murray A, Nelson S, Nicolaou A, Nirenberg A, Noble S, Nolan LS, Nus M, Van On C, Osei-Lah V, Peffers M, Palmer A, Palmer D, Palmer L, Parry-Smith W, Pawelec G, Peleg S, Perera R, Pitsillides A, Plack CJ, Progatzsky F, Pyott S, Rajput K, Rashid S, Ratnayaka JA, Ratnayake SAB, Rodriguez-Justo M, Rosa AC, Rule A, Sanger GJ, Sayers I, Saykin A, Selvarajah D, Sethi J, Shanahan C, Shen-Orr S, Sheridan C, Shiels P, Sidlauskas K, Sivaprasad S, Sluimer J, Small G, Smith P, Smith R, Snelling S, Spyridopoulos I, Srinivasa Raghavan R, Steel D, Steel KP, Stewart C, Stone K, Subbarayan S, Sussman M, Svensson J, Tadanki V, Tan AL, Tanzi RE, Tatler A, Tavares AAS, Tengku Mohd TAM, Tiganescu A, Timmons J, Tree J, Trivedi D, Tsochatzis EA, Tsimpida D, Vinke EJ, Whittaker A, Vallabh NA, Veighey K, Venables ZC, Reddy V, Vernooij MW, Verschoor C, Vinciguerra M, Vukanovic V, Vyazovskiy V, Walker J, Wakefield R, Watkins AJ, Webster A, Weight C, Weinberger B, Whitney SL, Willis R, Witkowski JM, Yeo LLL, Chung TY, Yu E, Zemel M, Calimport SRG and Bentley BL
Defining an Ageing-Related Pathology, Disease or Syndrome: International Consensus Statement
Short E, Adcock IM, Al-Sarireh B, Ager A, Ajjan R, Akbar N, Akeroyd MA, Alsaleh G, Al-Sharbatee G, Alavian K, Amoaku W, Andersen J, Antoniades C, Arends MJ, Astley S, Atan D, Attanoos R, Attems J, Bain S, Balaskas K, Balmus G, Bance M, Barber TM, Bardhan A, Barker K, Barnes P, Basatemur G, Bateman A, Bauer ME, Bellamy C, van Beek E, Bellantuono I, Benbow E, Bhandari S, Bhatnagar R, Bloom P, Bowdish D, Bowerman M, Burke M, Carare R, Carrington EV, Castillo-Quan JI, Clegg P, Cole J, Cota C, Chazot P, Chen C, Cheong Y, Christopher G, Church G, Clancy D, Cool P, Del Galdo F, Dalakoti M, Dasgupta S, Deane C, Dhasmana D, Dojcinov S, Di Prete M, Du H, Duggal NA, Ellmers T, Emanueli C, Emberton M, Erusalimsky JD, Feldmeyer L, Fleming A, Forbes K, Foster TC, Frasca D, Frayling I, Freedman D, Fülöp T, Ellison-Hughes G, Gazzard G, George C, Gil J, Glassock R, Goldin R, Green J, Guymer R, Haboubi H, Harries L, Hart S, Hartley D, Hasaballa S, Henein C, Helliwell M, Henderson E, Heer R, Holte K, Idris I, Isenburg D, Jylhävä J, Iqbal A, Jones SW, Kalaria R, Kanamarlapudi V, Kempf W, Kermack AJ, Kerns J, Koulman A, Khan AH, Kinross J, Klaucane K, Krishna Y, Gill HS, Lakatta E, Laconi E, Lazar A, Leeuwenburgh C, Leung S, Li X, van der Linde I, Lopes LV, Lorenzini A, Lotery A, Machado P, Mackie S, Madeddu P, Maier A, Mukkanna K, Manousou P, Markey O, Mauro C, McDonnell B, Medina RJ, Meran S, Metzler-Baddeley C, Meglinksi I, Milman N, Mitteldorf C, Montgomery R, Morris AC, Mühleisen B, Mukherkee A, Murray A, Nelson S, Nicolaou A, Nirenberg A, Noble S, Nolan LS, Nus M, Van On C, Osei-Lah V, Peffers M, Palmer A, Palmer D, Palmer L, Parry-Smith W, Pawelec G, Peleg S, Perera R, Pitsillides A, Plack CJ, Progatzsky F, Pyott S, Rajput K, Rashid S, Ratnayaka JA, Ratnayake SAB, Rodriguez-Justo M, Rosa AC, Rule A, Sanger GJ, Sayers I, Saykin A, Selvarajah D, Sethi J, Shanahan C, Shen-Orr S, Sheridan C, Shiels P, Sidlauskas K, Sivaprasad S, Sluimer J, Small G, Smith P, Smith R, Snelling S, Spyridopoulos I, Srinivasa Raghavan R, Steel D, Steel KP, Stewart C, Stone K, Subbarayan S, Sussman M, Svensson J, Tadanki V, Tan AL, Tanzi RE, Tatler A, Tavares AAS, Tengku Mohd TAM, Tiganescu A, Timmons J, Tree J, Trivedi D, Tsochatzis EA, Tsimpida D, Vinke EJ, Whittaker A, Vallabh NA, Veighey K, Venables ZC, Reddy V, Vernooij MW, Verschoor C, Vinciguerra M, Vukanovic V, Vyazovskiy V, Walker J, Wakefield R, Watkins AJ, Webster A, Weight C, Weinberger B, Whitney SL, Willis R, Witkowski JM, Yeo LLL, Chung TY, Yu E, Zemel M, Calimport SRG and Bentley BL
Around the world, individuals are living longer, but an increased average lifespan does not always equate to an increased healthspan. With advancing age, the increased prevalence of ageing-related diseases can have a significant impact on health status, functional capacity, and quality of life. It is therefore vital to develop comprehensive classification and staging systems for ageing-related pathologies, diseases and syndromes. This will allow societies to better identify, quantify, understand, and meet the healthcare, workforce, wellbeing, and socioeconomic needs of ageing populations, while supporting the development and utilisation of interventions to prevent or to slow, halt or reverse the progression of ageing-related pathologies.
How do neurons age? A focused review on the aging of the microtubular cytoskeleton
Richardson B, Goedert T, Quraishe S, Deinhardt K and Mudher A
How do neurons age? A focused review on the aging of the microtubular cytoskeleton
Richardson B, Goedert T, Quraishe S, Deinhardt K and Mudher A
Aging is the leading risk factor for Alzheimer's disease and other neurodegenerative diseases. We now understand that a breakdown in the neuronal cytoskeleton, mainly underpinned by protein modifications leading to the destabilization of microtubules, is central to the pathogenesis of Alzheimer's disease. This is accompanied by morphological defects across the somatodendritic compartment, axon, and synapse. However, knowledge of what occurs to the microtubule cytoskeleton and morphology of the neuron during physiological aging is comparatively poor. Several recent studies have suggested that there is an age-related increase in the phosphorylation of the key microtubule stabilizing protein tau, a modification, which is known to destabilize the cytoskeleton in Alzheimer's disease. This indicates that the cytoskeleton and potentially other neuronal structures reliant on the cytoskeleton become functionally compromised during normal physiological aging. The current literature shows age-related reductions in synaptic spine density and shifts in synaptic spine conformation which might explain age-related synaptic functional deficits. However, knowledge of what occurs to the microtubular and actin cytoskeleton, with increasing age is extremely limited. When considering the somatodendritic compartment, a regression in dendrites and loss of dendritic length and volume is reported whilst a reduction in soma volume/size is often seen. However, research into cytoskeletal change is limited to a handful of studies demonstrating reductions in and mislocalizations of microtubule-associated proteins with just one study directly exploring the integrity of the microtubules. In the axon, an increase in axonal diameter and age-related appearance of swellings is reported but like the dendrites, just one study investigates the microtubules directly with others reporting loss or mislocalization of microtubule-associated proteins. Though these are the general trends reported, there are clear disparities between model organisms and brain regions that are worthy of further investigation. Additionally, longitudinal studies of neuronal/cytoskeletal aging should also investigate whether these age-related changes contribute not just to vulnerability to disease but also to the decline in nervous system function and behavioral output that all organisms experience. This will highlight the utility, if any, of cytoskeletal fortification for the promotion of healthy neuronal aging and potential protection against age-related neurodegenerative disease. This review seeks to summarize what is currently known about the physiological aging of the neuron and microtubular cytoskeleton in the hope of uncovering mechanisms underpinning age-related risk to disease.
Holistic vibrational spectromics assessment of human cartilage for osteoarthritis diagnosis
Cook H, Crisford A, Bourdakos K, Dunlop D, Oreffo ROC and Mahajan S
Holistic vibrational spectromics assessment of human cartilage for osteoarthritis diagnosis
Cook H, Crisford A, Bourdakos K, Dunlop D, Oreffo ROC and Mahajan S
Osteoarthritis (OA) is the most common degenerative joint disease, presented as wearing down of articular cartilage and resulting in pain and limited mobility for 1 in 10 adults in the UK [Osteoarthr. Cartil.28(6), 792 (2020)10.1016/j.joca.2020.03.004]. There is an unmet need for patient friendly paradigms for clinical assessment that do not use ionizing radiation (CT), exogenous contrast enhancing dyes (MRI), and biopsy. Hence, techniques that use non-destructive, near- and shortwave infrared light (NIR, SWIR) may be ideal for providing label-free, deep tissue interrogation. This study demonstrates multimodal "spectromics", low-level abstraction data fusion of non-destructive NIR Raman scattering spectroscopy and NIR-SWIR absorption spectroscopy, providing an enhanced, interpretable "fingerprint" for diagnosis of OA in human cartilage. This is proposed as method level innovation applicable to both arthro- or endoscopic (minimally invasive) or potential exoscopic (non-invasive) optical approaches. Samples were excised from femoral heads post hip arthroplasty from OA patients (n = 13) and age-matched control (osteoporosis) patients (n = 14). Under multivariate statistical analysis and supervised machine learning, tissue was classified to high precision: 100% segregation of tissue classes (using 10 principal components), and a classification accuracy of 95% (control) and 80% (OA), using the combined vibrational data. There was a marked performance improvement (5 to 6-fold for multivariate analysis) using the spectromics fingerprint compared to results obtained from solely Raman or NIR-SWIR data. Furthermore, clinically relevant tissue components were identified through discriminatory spectral features - spectromics biomarkers - allowing interpretable feedback from the enhanced fingerprint. In summary, spectromics provides comprehensive information for early OA detection and disease stratification, imperative for effective intervention in treating the degenerative onset disease for an aging demographic. This novel and elegant approach for data fusion is compatible with various NIR-SWIR optical devices that will allow deep non-destructive penetration.
Classification of osteoarthritic and healthy cartilage using deep learning with Raman spectra
Kok YE, Crisford A, Parkes A, Venkateswaran S, Oreffo R, Mahajan S and Pound M
Classification of osteoarthritic and healthy cartilage using deep learning with Raman spectra
Kok YE, Crisford A, Parkes A, Venkateswaran S, Oreffo R, Mahajan S and Pound M
Raman spectroscopy is a rapid method for analysing the molecular composition of biological material. However, noise contamination in the spectral data necessitates careful pre-processing prior to analysis. Here we propose an end-to-end Convolutional Neural Network to automatically learn an optimal combination of pre-processing strategies, for the classification of Raman spectra of superficial and deep layers of cartilage harvested from 45 Osteoarthritis and 19 Osteoporosis (Healthy controls) patients. Using 6-fold cross-validation, the Multi-Convolutional Neural Network achieves comparable or improved classification accuracy against the best-performing Convolutional Neural Network applied to either the raw or pre-processed spectra. We utilised Integrated Gradients to identify the contributing features (Raman signatures) in the network decision process, showing they are biologically relevant. Using these features, we compared Artificial Neural Networks, Decision Trees and Support Vector Machines for the feature selection task. Results show that training on fewer than 3 and 300 features, respectively, for the disease classification and layer assignment task provide performance comparable to the best-performing CNN-based network applied to the full dataset. Our approach, incorporating multi-channel input and Integrated Gradients, can potentially facilitate the clinical translation of Raman spectroscopy-based diagnosis without the need for laborious manual pre-processing and feature selection.
Deaf awareness strategies in healthcare
Hough K, Tsimpida D, Boswell S, Satchwell C, Smith S, Dhuria P, Newberry E, Impey B, Hudson M and Newman T
Retinal peri-arteriolar versus peri-venular amyloidosis, hippocampal atrophy, and cognitive impairment: exploratory trial
Dumitrascu OM, Doustar J, Fuchs DT, Koronyo Y, Sherman DS, Miller MS, Johnson KO, Carare RO, Verdooner SR, Lyden PD, Schneider JA, Black KL and Koronyo-Hamaoui M
Retinal peri-arteriolar versus peri-venular amyloidosis, hippocampal atrophy, and cognitive impairment: exploratory trial
Dumitrascu OM, Doustar J, Fuchs DT, Koronyo Y, Sherman DS, Miller MS, Johnson KO, Carare RO, Verdooner SR, Lyden PD, Schneider JA, Black KL and Koronyo-Hamaoui M
The relationship between amyloidosis and vasculature in cognitive impairment and Alzheimer's disease (AD) pathogenesis is increasingly acknowledged. We conducted a quantitative and topographic assessment of retinal perivascular amyloid plaque (AP) distribution in individuals with both normal and impaired cognition. Using a retrospective dataset of scanning laser ophthalmoscopy fluorescence images from twenty-eight subjects with varying cognitive states, we developed a novel image processing method to examine retinal peri-arteriolar and peri-venular curcumin-positive AP burden. We further correlated retinal perivascular amyloidosis with neuroimaging measures and neurocognitive scores. Our study unveiled that peri-arteriolar AP counts surpassed peri-venular counts throughout the entire cohort (P < 0.0001), irrespective of the primary, secondary, or tertiary vascular branch location, with a notable increase among cognitively impaired individuals. Moreover, secondary branch peri-venular AP count was elevated in the cognitively impaired (P < 0.01). Significantly, peri-venular AP count, particularly in secondary and tertiary venules, exhibited a strong correlation with clinical dementia rating, Montreal cognitive assessment score, hippocampal volume, and white matter hyperintensity count. In conclusion, our exploratory analysis detected greater peri-arteriolar versus peri-venular amyloidosis and a marked elevation of amyloid deposition in secondary branch peri-venular regions among cognitively impaired subjects. These findings underscore the potential feasibility of retinal perivascular amyloid imaging in predicting cognitive decline and AD progression. Larger longitudinal studies encompassing diverse populations and AD-biomarker confirmation are warranted to delineate the temporal-spatial dynamics of retinal perivascular amyloid deposition in cognitive impairment and the AD continuum.
Longitudinal urinary neopterin is associated with hearing threshold change over time in independent older adults
Kidd RL, Agyemang-Prempeh A, Sanderson A, Stuart C, Mahajan S, Verschuur CA and Newman TA
Longitudinal urinary neopterin is associated with hearing threshold change over time in independent older adults
Kidd RL, Agyemang-Prempeh A, Sanderson A, Stuart C, Mahajan S, Verschuur CA and Newman TA
Low-grade chronic inflammation is associated with many age-related conditions. Non-invasive methods to monitor low-grade chronic inflammation may improve the management of older people at risk of poorer outcomes. This longitudinal cohort study has determined baseline inflammation using neopterin volatility in monthly urine samples of 45 independent older adults (aged 65-75 years). Measurement of neopterin, an inflammatory metabolite, enabled stratification of individuals into risk categories based on how often in a 12-month period their neopterin level was raised. Hearing was measured (pure-tone audiometry) at baseline, 1 year and 3 years of the study. Results show that those in the highest risk category (neopterin raised greater than 50% of the time) saw greater deterioration, particularly in high-frequency, hearing. A one-way Welch's ANOVA showed a significant difference between the risk categories for change in high-frequency hearing (W (3, 19.6) = 9.164, p = 0.0005). Despite the study size and duration individuals in the highest risk category were more than twice as likely to have an additional age-related morbidity than those in the lowest risk category. We conclude that volatility of neopterin in urine may enable stratification of those at greatest risk of progression of hearing loss.
Mild Systemic Inflammation Increases Erythrocyte Fragility
Stuart CM, Jacob C, Varatharaj A, Howard S, Chouhan JK, Teeling JL and Galea I
Mild Systemic Inflammation Increases Erythrocyte Fragility
Stuart CM, Jacob C, Varatharaj A, Howard S, Chouhan JK, Teeling JL and Galea I
There is growing evidence that inflammation impairs erythrocyte structure and function. We assessed the impact of mild systemic inflammation on erythrocyte fragility in three different settings. In order to investigate causation, erythrocyte osmotic fragility was measured in mice challenged with a live attenuated bacterial strain to induce low-grade systemic inflammation; a significant increase in erythrocyte osmotic fragility was observed. To gather evidence that systemic inflammation is associated with erythrocyte fragility in humans, two observational studies were conducted. First, using a retrospective study design, the relationship between reticulocyte-based surrogate markers of haemolysis and high-sensitivity C-reactive protein was investigated in 9292 healthy participants of the UK Biobank project. Secondly, we prospectively assessed the relationship between systemic inflammation (measured by the urinary neopterin/creatinine ratio) and erythrocyte osmotic fragility in a mixed population (n = 54) of healthy volunteers and individuals with long-term medical conditions. Both human studies were in keeping with a relationship between inflammation and erythrocyte fragility. Taken together, we conclude that mild systemic inflammation increases erythrocyte fragility and may contribute to haemolysis. Further research is needed to assess the molecular underpinnings of this pathway and the clinical implications in inflammatory conditions.
Is CAA a perivascular brain clearance disease? A discussion of the evidence to date and outlook for future studies
van Veluw SJ, Benveniste H, Bakker ENTP, Carare RO, Greenberg SM, Iliff JJ, Lorthois S, Van Nostrand WE, Petzold GC, Shih AY and van Osch MJP
Is CAA a perivascular brain clearance disease? A discussion of the evidence to date and outlook for future studies
van Veluw SJ, Benveniste H, Bakker ENTP, Carare RO, Greenberg SM, Iliff JJ, Lorthois S, Van Nostrand WE, Petzold GC, Shih AY and van Osch MJP
The brain's network of perivascular channels for clearance of excess fluids and waste plays a critical role in the pathogenesis of several neurodegenerative diseases including cerebral amyloid angiopathy (CAA). CAA is the main cause of hemorrhagic stroke in the elderly, the most common vascular comorbidity in Alzheimer's disease and also implicated in adverse events related to anti-amyloid immunotherapy. Remarkably, the mechanisms governing perivascular clearance of soluble amyloid β-a key culprit in CAA-from the brain to draining lymphatics and systemic circulation remains poorly understood. This knowledge gap is critically important to bridge for understanding the pathophysiology of CAA and accelerate development of targeted therapeutics. The authors of this review recently converged their diverse expertise in the field of perivascular physiology to specifically address this problem within the framework of a Leducq Foundation Transatlantic Network of Excellence on Brain Clearance. This review discusses the overarching goal of the consortium and explores the evidence supporting or refuting the role of impaired perivascular clearance in the pathophysiology of CAA with a focus on translating observations from rodents to humans. We also discuss the anatomical features of perivascular channels as well as the biophysical characteristics of fluid and solute transport.
Correlative geochemical imaging of Desmophyllum dianthus reveals biomineralisation strategy as a key coral vital effect
Standish CD, Trend J, Kleboe J, Chalk TB, Mahajan S, Milton JA, Page TM, Robinson LF, Stewart JA and Foster GL
Correlative geochemical imaging of Desmophyllum dianthus reveals biomineralisation strategy as a key coral vital effect
Standish CD, Trend J, Kleboe J, Chalk TB, Mahajan S, Milton JA, Page TM, Robinson LF, Stewart JA and Foster GL
The chemical and isotopic composition of stony coral skeletons form an important archive of past climate. However, these reconstructions are largely based on empirical relationships often complicated by "vital effects" arising from uncertain physiological processes of the coral holobiont. The skeletons of deep-sea corals, such as Desmophyllum dianthus, are characterised by micron-scale or larger geochemical heterogeneity associated with: (1) centres of calcification (COCs) where nucleation of new skeleton begins, and (2) fibres that thicken the skeleton. These features are difficult to sample cleanly using traditional techniques, resulting in uncertainty surrounding both the causes of geochemical differences and their influence on environmental signals. Here we combine optical, and in-situ chemical and isotopic, imaging tools across a range of spatial resolutions (~ 100 nm to 10 s of μm) in a correlative multimodal imaging (CMI) approach to isolate the microstructural geochemistry of each component. This reveals COCs are characterised by higher organic content, Mg, Li and Sr and lower U, B and δB compared to fibres, reflecting the contrasting biomineralisation mechanisms employed to construct each feature. CMI is rarely applied in Environmental/Earth Sciences, but here we illustrate the power of this approach to unpick the "vital effects" in D. dianthus, and by extension, other scleractinian corals.
Shaping the future of preclinical development of successful disease-modifying drugs against Alzheimer's disease: a systematic review of tau propagation models
Basheer N, Buee L, Brion JP, Smolek T, Muhammadi MK, Hritz J, Hromadka T, Dewachter I, Wegmann S, Landrieu I, Novak P, Mudher A and Zilka N
Shaping the future of preclinical development of successful disease-modifying drugs against Alzheimer's disease: a systematic review of tau propagation models
Basheer N, Buee L, Brion JP, Smolek T, Muhammadi MK, Hritz J, Hromadka T, Dewachter I, Wegmann S, Landrieu I, Novak P, Mudher A and Zilka N
The transcellular propagation of the aberrantly modified protein tau along the functional brain network is a key hallmark of Alzheimer's disease and related tauopathies. Inoculation-based tau propagation models can recapitulate the stereotypical spread of tau and reproduce various types of tau inclusions linked to specific tauopathy, albeit with varying degrees of fidelity. With this systematic review, we underscore the significance of judicious selection and meticulous functional, biochemical, and biophysical characterization of various tau inocula. Furthermore, we highlight the necessity of choosing suitable animal models and inoculation sites, along with the critical need for validation of fibrillary pathology using confirmatory staining, to accurately recapitulate disease-specific inclusions. As a practical guide, we put forth a framework for establishing a benchmark of inoculation-based tau propagation models that holds promise for use in preclinical testing of disease-modifying drugs.
Patient and public involvement and engagement (PPIE): how valuable and how hard? An evaluation of ALL_EARS@UoS PPIE group, 18 months on
Hough K, Grasmeder M, Parsons H, Jones WB, Smith S, Satchwell C, Hobday I, Taylor S and Newman T
Patient and public involvement and engagement (PPIE): how valuable and how hard? An evaluation of ALL_EARS@UoS PPIE group, 18 months on
Hough K, Grasmeder M, Parsons H, Jones WB, Smith S, Satchwell C, Hobday I, Taylor S and Newman T
ALL_EARS@UoS is a patient and public involvement and engagement (PPIE) group for people with lived experience of hearing loss. The purpose of the group is to share experiences of hearing loss and hearing healthcare, inform research and improve services for patients at University of Southampton Auditory Implant Service. A year after inception, we wanted to critically reflect on the value and challenges of the group. Four members of ALL_EARS@UoS were recruited to an evaluation steering group. This paper reports the evaluation of the group using the UK Standards for Public Involvement.
Double-Clad Antiresonant Hollow-Core Fiber and Its Comparison with Other Fibers for Multiphoton Micro-Endoscopy
Szwaj M, Davidson IA, Johnson PB, Jasion G, Jung Y, Sandoghchi SR, Herdzik KP, Bourdakos KN, Wheeler NV, Mulvad HC, Richardson DJ, Poletti F and Mahajan S
Double-Clad Antiresonant Hollow-Core Fiber and Its Comparison with Other Fibers for Multiphoton Micro-Endoscopy
Szwaj M, Davidson IA, Johnson PB, Jasion G, Jung Y, Sandoghchi SR, Herdzik KP, Bourdakos KN, Wheeler NV, Mulvad HC, Richardson DJ, Poletti F and Mahajan S
Label-free and multiphoton micro-endoscopy can transform clinical histopathology by providing an in situ tool for diagnostic imaging and surgical treatment in diseases such as cancer. Key to a multiphoton imaging-based micro-endoscopic device is the optical fiber, for distortion-free and efficient delivery of ultra-short laser pulses to the sample and effective signal collection. In this work, we study a new hollow-core (air-filled) double-clad anti-resonant fiber (DC-ARF) as a high-performance candidate for multiphoton micro-endoscopy. We compare the fiber characteristics of the DC-ARF with a single-clad anti-resonant fiber (SC-ARF) and a solid core fiber (SCF). In this work, while the DC-ARF and the SC-ARF enable low-loss (<0.2 dBm), close to dispersion-free excitation pulse delivery (2000% of pulse width increase at 900 nm per 1 m fiber). An ideal optical fiber endoscope needs to be several meters long and should enable both excitation and collection through the fiber. Therefore, we performed multiphoton imaging on endoscopy-compatible 1 m and 3 m lengths of fiber in the back-scattered geometry, wherein the signals were collected either directly (non-descanned detection) or through the fiber (descanned detection). Second harmonic images were collected from barium titanate crystals as well as from biological samples (mouse tail tendon). In non-descanned detection conditions, the ARFs outperformed the SCF by up to 10 times in terms of signal-to-noise ratio of images. Significantly, only the DC-ARF, due to its high numerical aperture (NA) of 0.45 and wide-collection bandwidth (>1 µm), could provide images in the de-scanned detection configuration desirable for endoscopy. Thus, our systematic characterization and comparison of different optical fibers under different image collection configurations, confirms and establishes the utility of DC-ARFs for high-performing label-free multiphoton imaging-based micro-endoscopy.
Leveraging real-world data to improve cochlear implant outcomes: Is the data available?
Findlay C, Edwards M, Hough K, Grasmeder M and Newman TA
Leveraging real-world data to improve cochlear implant outcomes: Is the data available?
Findlay C, Edwards M, Hough K, Grasmeder M and Newman TA
A small but persistent proportion of individuals do not gain the expected benefit from cochlear implants(CI). A step-change in the understanding of factors affecting outcomes could come through data science. This study evaluates clinical data capture to assess the quality and utility of CI user's health records for data science, by assessing the recording of otitis media. Otitis media was selected as it is associated with the development of sensorineural hearing loss and may affect cochlear implant outcomes.
A laser-induced mouse model of progressive retinal degeneration with central sparing displays features of parafoveal geographic atrophy
Khan AH, Soundara Pandi SP, Scott JA, Sánchez-Bretaño A, Lynn SA, Ratnayaka JA, Teeling JL and Lotery AJ
A laser-induced mouse model of progressive retinal degeneration with central sparing displays features of parafoveal geographic atrophy
Khan AH, Soundara Pandi SP, Scott JA, Sánchez-Bretaño A, Lynn SA, Ratnayaka JA, Teeling JL and Lotery AJ
There are no disease-modifying treatments available for geographic atrophy (GA), the advanced form of dry age-related macular degeneration. Current murine models fail to fully recapitulate the features of GA and thus hinder drug discovery. Here we describe a novel mouse model of retinal degeneration with hallmark features of GA. We used an 810 nm laser to create a retinal lesion with central sparing (RLCS), simulating parafoveal atrophy observed in patients with progressive GA. Laser-induced RLCS resulted in progressive GA-like pathology with the development of a confluent atrophic lesion. We demonstrate significant changes to the retinal structure and thickness in the central unaffected retina over a 24-week post-laser period, confirmed by longitudinal optical coherence tomography scans. We further show characteristic features of progressive GA, including a gradual reduction in the thickness of the central, unaffected retina and of total retinal thickness. Histological changes observed in the RLCS correspond to GA pathology, which includes the collapse of the outer nuclear layer, increased numbers of GFAP + , CD11b + and FcγRI + cells, and damage to cone and rod photoreceptors. We demonstrate a laser-induced mouse model of parafoveal GA progression, starting at 2 weeks post-laser and reaching confluence at 24 weeks post-laser. This 24-week time-frame in which GA pathology develops, provides an extended window of opportunity for proof-of-concept evaluation of drugs targeting GA. This time period is an added advantage compared to several existing models of geographic atrophy.
Perfusion Imaging and Inflammation Biomarkers Provide Complementary Information in Alzheimer's Disease
Michopoulou S, Prosser A, Dickson J, Guy M, Teeling JL and Kipps C
Perfusion Imaging and Inflammation Biomarkers Provide Complementary Information in Alzheimer's Disease
Michopoulou S, Prosser A, Dickson J, Guy M, Teeling JL and Kipps C
Single photon emission tomography (SPECT) can detect early changes in brain perfusion to support the diagnosis of dementia. Inflammation is a driver for dementia progression and measures of inflammation may further support dementia diagnosis.
Tau-mediated axonal degeneration is prevented by activation of the Wld pathway
Stubbs K, Batchelor B, Sivanantharajah L, Sealey M, Ramirez-Moreno M, Ruiz E, Richardson B, Perry VH, Newman TA and Mudher A
Tau-mediated axonal degeneration is prevented by activation of the Wld pathway
Stubbs K, Batchelor B, Sivanantharajah L, Sealey M, Ramirez-Moreno M, Ruiz E, Richardson B, Perry VH, Newman TA and Mudher A
Tauopathy is characterized by neuronal dysfunction and degeneration occurring as a result of changes to the microtubule-associated protein tau. The neuronal changes evident in tauopathy bear striking morphological resemblance to those reported in models of Wallerian degeneration. The mechanisms underpinning Wallerian degeneration are not fully understood although it can be delayed by the expression of the slow Wallerian degeneration (Wld) protein, which has also been demonstrated to delay axonal degeneration in some models of neurodegenerative disease. Given the morphological similarities between tauopathy and Wallerian degeneration, this study investigated whether tau-mediated phenotypes can be modulated by co-expression of Wld. In a model of tauopathy in which expression of human 0N3R tau protein leads to progressive age-dependent phenotypes, Wld was expressed with and without activation of the downstream pathway. The olfactory receptor neuron circuit was used for these studies in adults, and the larval motor neuron system was employed in larvae. Tau phenotypes studied included neurodegeneration, axonal transport, synaptic deficits and locomotor behaviour. Impact on total tau was ascertained by assessing total, phosphorylated and misfolded tau levels by immunohistochemistry. Activation of the pathway downstream of Wld completely suppressed tau-mediated degeneration. This protective effect was evident even if the pathway downstream of Wld was activated several weeks after tau-mediated degeneration had become established. Though total tau levels were not altered, the protected neurons displayed significantly reduced MC1 immunoreactivity suggestive of clearance of misfolded tau, as well as a trend for a decline in tau species phosphorylated at the AT8 and PHF1 epitopes. In contrast, Wld expression without activation of the downstream protective pathway did not rescue tau-mediated degeneration in adults or improve tau-mediated neuronal dysfunction including deficits in axonal transport, synaptic alterations and locomotor behaviour in tau-expressing larvae. This collectively implies that the pathway mediating the protective effect of Wld intersects with the mechanism(s) of degeneration initiated by tau and can effectively halt tau-mediated degeneration at both early and late stages. Understanding the mechanisms underpinning this protection could identify much-needed disease-modifying targets for tauopathies.
Age-related changes in tau and autophagy in human brain in the absence of neurodegeneration
Chatterjee S, Sealey M, Ruiz E, Pegasiou CM, Brookes K, Green S, Crisford A, Duque-Vasquez M, Luckett E, Robertson R, Richardson P, Vajramani G, Grundy P, Bulters D, Proud C, Vargas-Caballero M and Mudher A
Age-related changes in tau and autophagy in human brain in the absence of neurodegeneration
Chatterjee S, Sealey M, Ruiz E, Pegasiou CM, Brookes K, Green S, Crisford A, Duque-Vasquez M, Luckett E, Robertson R, Richardson P, Vajramani G, Grundy P, Bulters D, Proud C, Vargas-Caballero M and Mudher A
Tau becomes abnormally hyper-phosphorylated and aggregated in tauopathies like Alzheimers disease (AD). As age is the greatest risk factor for developing AD, it is important to understand how tau protein itself, and the pathways implicated in its turnover, change during aging. We investigated age-related changes in total and phosphorylated tau in brain samples from two cohorts of cognitively normal individuals spanning 19-74 years, without overt neurodegeneration. One cohort utilised resected tissue and the other used post-mortem tissue. Total soluble tau levels declined with age in both cohorts. Phosphorylated tau was undetectable in the post-mortem tissue but was clearly evident in the resected tissue and did not undergo significant age-related change. To ascertain if the decline in soluble tau was correlated with age-related changes in autophagy, three markers of autophagy were tested but only two appeared to increase with age and the third was unchanged. This implies that in individuals who do not develop neurodegeneration, there is an age-related reduction in soluble tau which could potentially be due to age-related changes in autophagy. Thus, to explore how an age-related increase in autophagy might influence tau-mediated dysfunctions in vivo, autophagy was enhanced in a Drosophila model and all age-related tau phenotypes were significantly ameliorated. These data shed light on age-related physiological changes in proteins implicated in AD and highlights the need to study pathways that may be responsible for these changes. It also demonstrates the therapeutic potential of interventions that upregulate turnover of aggregate-prone proteins during aging.
The RESIST Study: Examining Cognitive Change in Rheumatoid Arthritis Patients with Mild Cognitive Impairment Being Treated with a TNF-Inhibitor Compared to a Conventional Synthetic Disease-Modifying Anti-Rheumatic Drug
Marr C, McDowell B, Holmes C, Edwards CJ, Cardwell C, McHenry M, Meenagh G, Teeling JL and McGuinness B
The RESIST Study: Examining Cognitive Change in Rheumatoid Arthritis Patients with Mild Cognitive Impairment Being Treated with a TNF-Inhibitor Compared to a Conventional Synthetic Disease-Modifying Anti-Rheumatic Drug
Marr C, McDowell B, Holmes C, Edwards CJ, Cardwell C, McHenry M, Meenagh G, Teeling JL and McGuinness B
Evidence suggests that TNF inhibitors (TNFi) used to treat rheumatoid arthritis (RA) may protect against Alzheimer's disease progression by reducing inflammation.
Biomarkers of Inflammation Increase with Tau and Neurodegeneration but not with Amyloid-β in a Heterogenous Clinical Cohort
Michopoulou S, Prosser A, Kipps C, Dickson J, Guy M and Teeling J
Biomarkers of Inflammation Increase with Tau and Neurodegeneration but not with Amyloid-β in a Heterogenous Clinical Cohort
Michopoulou S, Prosser A, Kipps C, Dickson J, Guy M and Teeling J
Neuroinflammation is an integral part of Alzheimer's disease (AD) pathology. Inflammatory mediators can exacerbate the production of amyloid-β (Aβ), the propagation of tau pathology and neuronal loss.